Part 2 of 2 Parts (Please read Part 1 first)
AI can help narrow the range of candidate materials for testing, characterize them based on their properties, and carry out real-time monitoring of those installed in fusion reactors. These capabilities allow the rapid screening and development of radiation-tolerant materials, reducing reliance on current time-intensive approaches.
AI also offers improved control of the plasma in fusion reactors. As discussed above, a key challenge in magnetic confinement fusion is to shape and maintain the high-temperature plasma within the fusion reactor.
However, the plasmas in these fusion reactors are inherently unstable. A control system needs to coordinate the tokamak’s many magnets, and adjust their voltage thousands of times per second to prevent the plasma from touching the walls of the vessel. This could lead to the loss of heat and potentially damage the materials inside the fusion reactor.
Researchers from the U.K.-based company Google DeepMind have utilized a form of AI called deep reinforcement learning to keep the plasma stable and to accurately sculpt it into different shapes. This allows researchers to understand how the plasma behaves under different conditions.
A team at Princeton University in the U.S. has also used deep reinforcement learning to forecast disturbances in fusion plasma known as “tearing mode instabilities,” up to three hundred milliseconds before they occur. Tearing instabilities are a major form of disruption that can occur, halting the fusion process. They happen when the magnetic field lines within a plasma break and create an opportunity for that plasma to escape the control system in a fusion reactor.
Work at the U.K. Atomic Energy Authority (UKAEA) is addressing critical challenges in materials performance and structural integrity by integrating a variety of techniques. These include machine learning models, for evaluating what’s known as the residual stress of materials. Residual stress is a way to measure performance that’s locked into materials during manufacturing or operation. It can seriously affect the reliability and safety of fusion reactor components under extreme conditions.
A key outcome of this work is the development of a technique that integrates data from experiments with a machine learning-powered predictive model to evaluate residual stress in fusion components.
This new technique has been validated through collaborations with leading institutions, including the National Physical Laboratory and UKAEA’s materials research facility. These developments provide efficient and accurate assessments of materials performance and have redefined the evaluation of residual stress. They have unlocked new possibilities for assessing the structural integrity of components used in fusion reactors.
This research directly supports the European Demonstration Power Plant (EU-DEMO) and the Spherical Tokamak for Energy Production (STEP) projects. These projects aim to deliver a demonstration fusion power plant and prototype fusion power plant, respectively, to scale. Their success depends on maintaining the structural integrity of critical components under extreme conditions.
By using many AI-based approaches in a coordinated way, scientists can ensure that fusion reactors are physically robust and economically viable, accelerating the path to commercialization. AI can be used to develop accurate simulations of fusion reactors that integrate insights from plasma physics, materials science, engineering, and other aspects of the process. By simulating fusion systems within these virtual environments, scientists can optimize reactor design and operational strategies.
Spherical Tokamak for Energy Production
Nuclear Fusion 109 – Artificial Intelligence And Nuclear Fusion – Part 2 of 2 Parts
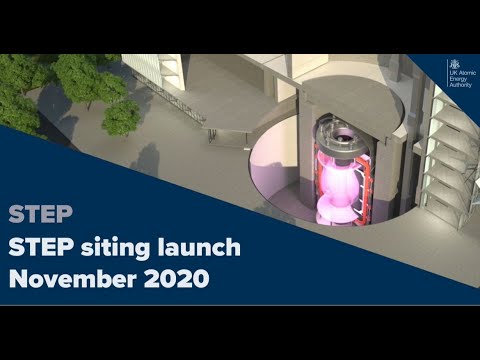